
The social network faces more accusations of favouring images based on certain races, genders, religions and ages. Are these machine learning biases intentional, or an accidental bi-product of skewed training data?
Serious flaws found in Twitter’s automated image-cropping programme, which favoured white people over those with darker skin colour, sent ripples across the internet last year.
Further evidence reveals that its algorithm is not only “racist”. It also apparently favours younger people, non-Muslims, and the able-bodied.
We’ve said it before and we’ll say it again. Artificial intelligence is there to augment human capabilities. Intelligent automation speeds up functionalities and improves efficiency. But in many instances, such as Twitter’s photo-editing system, there can be a wider social impact.
Take your Pick
The findings, revealed last year, led to an explosion on social media. Users took to the platform to publicly test the coding behaviour with various images (from celebrities to cartoon characters) in order to see what the results appeared. They ended up calling it a ‘horrible experiment’.
Twitter apologised for the embarrassing technical testing blunder at the time, accepting that it didn’t go far enough in testing the service for bias before they started using it. The algorithm, trained on human eye-tracking data, works by estimating what a person might want to see first within a picture. This is then cropped to an easily viewable size, according to Twitter.
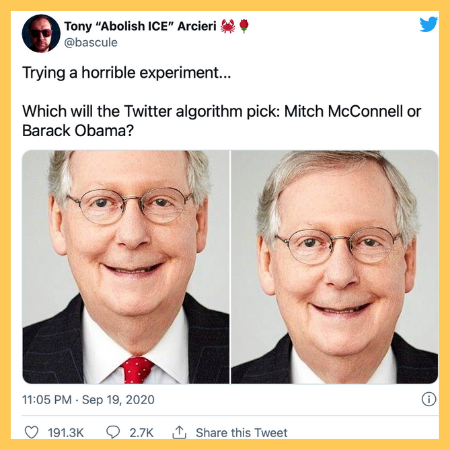


However, this month it faced fresh criticism that its image cropping programmes are also coded with implicit bias against ethnic and social minorities.
The tech giant claimed to have already started phasing out the older system, which it initially reported to only be a problem on mobile versions of the app. They accepted the allegations but also argued that the issues were due to several complicated factors.
Stats Don’t Lie
Recently published statistics released by Twitter found:
- An 8% difference in favour of women over men
- A 4% difference favouring white people over black people
- A 7% difference favouring white women over black women
- A 2% difference in favour of white men over black men
“We considered the trade-offs between the speed and consistency of automated cropping with the potential risks we saw in this research,” wrote Rumman Chowdhury, Twitter’s director of software engineering.
“One of our conclusions is that not everything on Twitter is a good candidate for an algorithm, and in this case, how to crop an image is a decision best made by people,” she said.
Algorithms aren’t Always the Answer
At a recent Def Con hacker conference in Las Vegas, the social platform announced that it was an unintentional, but very important body of research. The specific technology has largely been decommissioned.
The racial bias was embarrassing for Twitter, but it is also not completely unheard of in machine learning. AI systems trained by existing data resources have become imbued with existing biases in society.
Like the photo-cropping algorithm, when companies train artificial intelligence to learn from their users’ behaviour the AI systems can internalise prejudices that they would never intentionally write into a program. So the fact that people wearing religious headscarves, pictured in wheelchairs or if they have grey hair are not favoured in Twitter’s cropping programme, is not a result of “pre-meditated” calculated coding.
A Human Touch
Not everything can be solved by an algorithm. Human functions can never, and will never be fully replaced by technology.
Take the space OVERWRITE.ai operates in – real estate. The raison d’etre of PropTech is NOT to make realtors obsolete. Real estate needs real agents. There are too many emotional nuances that make up the sales journey which technology could never predict or replace.
If technology can remove any potential for error however, (take the automatic spellchecking feature of OVERWRITE.ai, for example, which is built into the description-writing code) there is clearly a time-saving advantage.
Our logic is simple. If we solve a problem by increasing efficiency for the end-user, we enable them to focus their time on higher value tasks. By no longer having to worry about embarrassing spelling errors or unengaging copy, our estate agents can focus more time serving their clients, and closing more sales.
The Social Code
Twitter has vowed to continue to share what it learns, and will open-source its analysis so others can review and learn from improvements to any of its automated programmes.
As social media consumption continues to grow at drastically fast rates, we can only hope the network giants will deliver as originally intended; to connect people within a socially-inclusive and unprejudiced, digital landscape.
But like any new or modern technology, we have to trust that those behind it are aware of the potential risks to any automated programming. Additionally, continual investment in research, in whatever industry, should always be implemented to ensure the end-user gets the best possible product.
In the end, intelligent automation is still a human construct. We’re teaching machines to learn the way we do. And we learn by trial and error. So why expect any different from the machines we are teaching?
OVERWRITE.ai is a world first, user-friendly marketing solution that allows estate agents to instantly autowrite unique, search-optimised property descriptions, taking the boring out of the daily grind.
For informative and light-hearted news and views on the world of real estate, follow OVERWRITE.ai on Instagram and LinkedIn, and keep up-to-date with our weekly NewsBites blog.